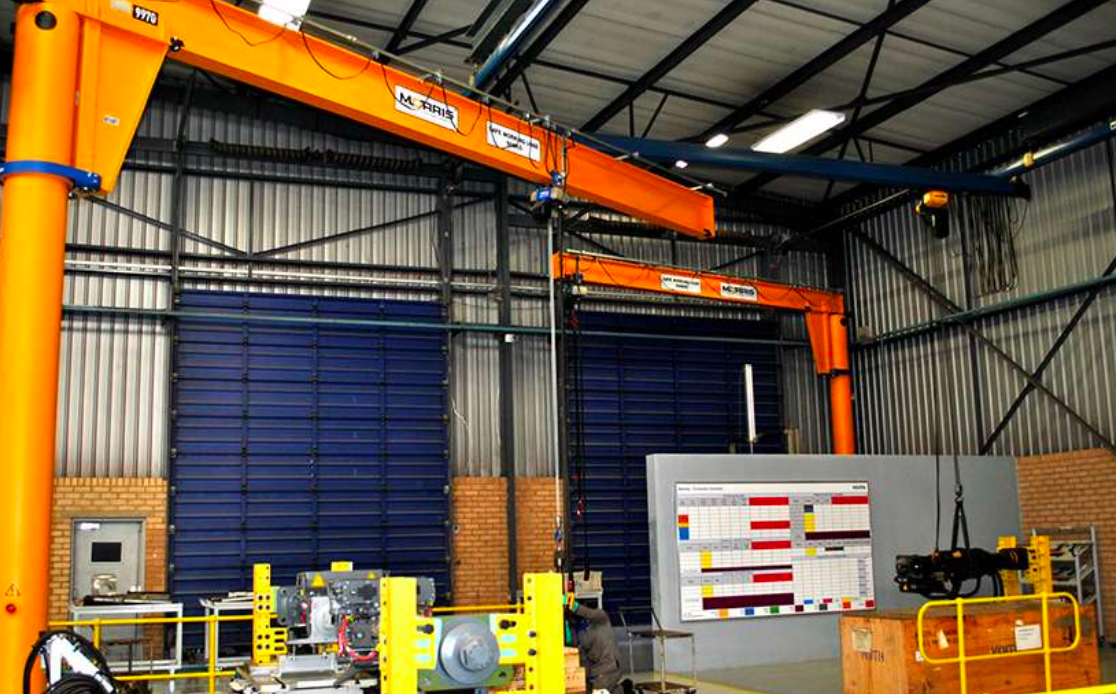
Features
Manufacturer C has two ‘configure to order’ (CTO) products and wanted to know which configurations of features are the most popular and which features, or combination of features, are the most significant in defining those groups.
Data was extracted from the core manufacturing application for the prior year. This resulted in a very narrow data set of three columns consisting of reference number, feature and a value for that feature.
“Data related to Usage Data, Maintenance Data, Asset Data, all add up to making the new age Production cycles insights driven”
Next the cross-reference data was needed to define which reference ID belonged to which product as the same feature was applicable to either product type. As the final data set extracted was with a list of features, during discussions the question arose – “Are there any features we have never used in production?”.
Initial exploration showed which features were the most common and indeed there were several features which had never, or rarely, been selected. Useful feedback for R&D for future product development.
As is usual we ran into some unforeseen issues, such as, if multiple of a configuration were purchased on the same order there was only one configuration held with multiple production orders. Data had to be manipulated to duplicate the features data with a synthetic number added to the reference.
Next the data was transformed into two data sets, one per product, which could be used by the clustering and weighting algorithms. A value was assigned for every feature. For each reference number, values were either as a binary, a simple Yes or No, or ordinal, i.e. data was put into a number of categories. Having transformed the data, a number of clustering algorithms was applied to each product data set separately.
What was striking was that one product had a small number of tightly grouped clusters whilst the other product had a higher number of more loosely grouped clusters. When this was presented to the business it was clear that their expectation was that there would be a certain feature, that would be the dominant one in defining the clusters. Unfortunately, this was not to be the case, that feature wasn’t significant in any cluster. Gut feel and perceived wisdom had been shown not to prevail.
Process
Having established the clusters the next question arose which was does any combination of features cause longer production times and if so in which area. Secondary question was to compare actual against estimated times for each step in the process. This was to be done for the product with the wider spread of clusters.
Process mining was used to see how each product went through production. Initial results were inconclusive except that the number of exceptions was too high. Other features were added which helped to highlight which configuration of product was likely to be delayed or have to be reworked. Key findings were deviation from quoted features and availability of components between factories.
Departure Board
Final step was to combine numerous pieces of data to create a daily summary of build orders and then assign likelihoods as to why it would be delayed. Aim was to allow management to focus on resolving the issues. As with most adaptations the only way to achieve this was as a separate application, as to do it “by the book” would take too long and cost more.